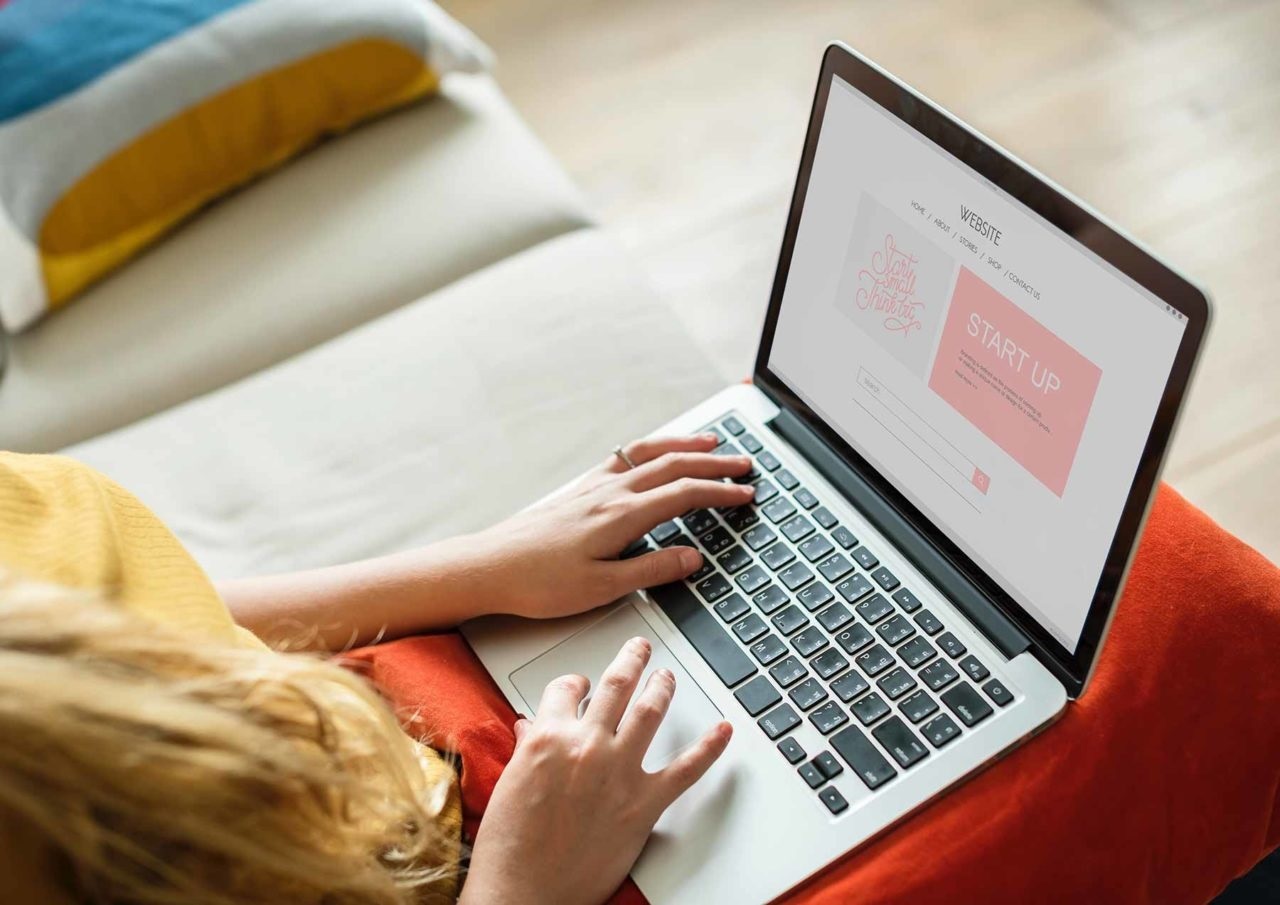
A/B testing is a pivotal technique for businesses to make data-informed decisions and effectively optimise their products or services. This guide offers an extensive overview of A/B testing, covering its principles, applications, best practices, tools, challenges, and implementation in a data science course. It also concludes with its significance in contemporary business strategies.
- Understanding A/B Testing
A/B testing, often referred to as split testing, is a method for comparing two or more forms of a webpage, email, app feature, or marketing campaign. The main objective is to assess which version performs better in achieving specific goals, such as boosting conversion rates, click-through rates, or revenue. The “A/B testing” method serves as the control group, while the “B testing” version includes a specific variation. By measuring user responses to each version, businesses can make informed decisions about which variant to implement.
- Applications of A/B Testing
The versatility of A/B testing extends across various domains:
- Website Optimisation: Businesses use A/B testing to optimise website elements like layouts, headlines, and call-to-action buttons to improve user engagement and conversion rates.
- Email Marketing: A/B testing is instrumental in testing subject lines, email content, and sending times to maximise open rates and click-through rates.
- Product Development: A/B testing enables organisations to experiment with new features, user interfaces, and pricing strategies to improve product usability and customer satisfaction.
- The A/B Testing Process
Executing a successful A/B test involves several key steps:
- Define Goals: Clearly define the objectives of the A/B test, such as increasing sales, sign-ups, or engagement metrics.
- Create Variations: Develop multiple variations of the element being tested, ensuring that each version differs only in the specific change under evaluation.
- Randomize and Split Traffic: Randomly assign users or visitors to each variation group to ensure unbiased results and statistical validity.
- Measure Performance: Collect data on key metrics for each variation group and analyse the results to determine the effectiveness of each variant.
- Best Practices for A/B Testing
Adhering to best practices is crucial for obtaining accurate and actionable insights from A/B tests:
- Test One Variable at a Time: Focus on testing one variable or change at a time to isolate its impact and avoid confounding variables.
- Collect Sufficient Data: Ensure that the sample size for each variation group is large enough to yield statistically significant results and minimise the risk of false positives.
- Run Tests Concurrently: Conduct A/B tests concurrently to mitigate the influence of various external factors and seasonality on the results.
- Document and Analyse Results: Document the test setup, hypothesis, and outcomes to facilitate analysis and inform future decision-making.
- Tools and Platforms for A/B Testing
Several tools and platforms facilitate the execution of A/B tests, including:
- Google Optimize: A free tool that allows users to conduct A/B tests directly within Google Analytics, providing access to advanced targeting options and real-time analytics.
- Optimizely: A popular A/B testing platform known for its user-friendly interface, robust feature set, and comprehensive optimisation capabilities.
- VWO (Visual Website Optimizer): A versatile optimisation platform that enables users to create, launch, and analyse A/B tests across websites, mobile apps, and email campaigns.
- Challenges of A/B Testing
Despite its benefits, A/B testing presents several challenges:
- Duration and Sample Size: A/B tests may require significant time and traffic volume to yield statistically significant results, especially for subtle changes or small effects.
- Risk of Bias: Biases in sample selection, test setup, or interpretation of results can skew the outcomes of A/B tests and lead to erroneous conclusions.
- Resource Intensiveness: Designing, executing, and analysing A/B tests demands resources, including time, personnel, and technology, which may pose challenges for some organisations.
- Implementing A/B Testing in Data Science Courses
Integrating A/B testing into a data science course provides students with useful and practical experience in experimental design, hypothesis testing, and data analysis. Hands-on projects and case studies involving A/B testing equip students with valuable analytical skills and prepare them for real-world applications in business and industry.
- Conclusion
In today’s data-driven business landscape, A/B testing is pivotal for informed decision-making and continuous improvement. By embracing best practices, leveraging appropriate tools, and understanding the nuances of experimental design, individuals trained in a data science course in Pune can harness the power of A/B testing to drive organisational success and achieve strategic objectives.
Business Name: ExcelR – Data Science, Data Analyst Course Training
Address: 1st Floor, East Court Phoenix Market City, F-02, Clover Park, Viman Nagar, Pune, Maharashtra 411014
Phone Number: 096997 53213
Email Id: enquiry@excelr.com